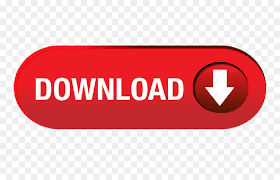

However, it remains necessary to determine which features extracted by these systems and which classification models are appropriate for our task. word tokens, parts of speech tags) from natural language text. More generally, existing NLP systems can extract pre-specified features (e.g. For example, we sought to enable document search for abnormalities in broad ear regions for which highly granular labels are unnecessary. Additionally, the granularity of the ontology may be inappropriate for the use case. The size and complexity of such ontologies places a potentially prohibitive burden on the labeler, typically a physician or study staff member, who is required to learn at least part of the ontology in order to perform the annotation task.
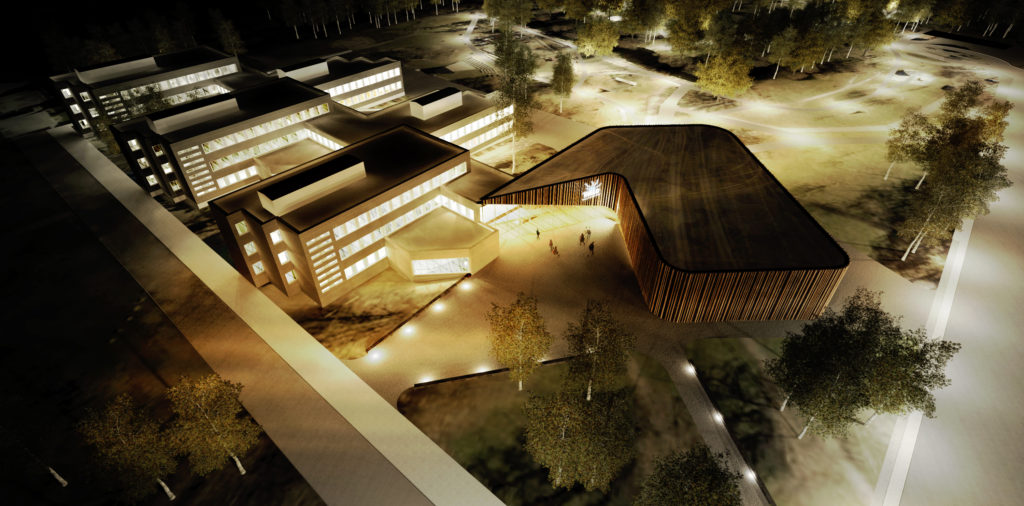
This requires training documents to be labeled with detailed concepts from the standardized ontology utilized by the system (e.g. Although significant progress has been made in the biomedical domain toward the development of such systems for text analysis, fine-tuning is usually necessary to achieve acceptable performance for specific use cases. enlarged) identified in each report to be used for search indexing. vestibular aqueduct) and attributes (e.g. The system would generate labels that correspond to entities (e.g. Ideally, we would like to utilize a fully automated knowledge extraction system for which it would be necessary only to supply radiology reports. Therefore, to facilitate the effective use of anatomic information contained in radiology reports for audiology research, we adopted a machine learning procedure. As shown in the work presented here, these approaches lack sensitivity (recall) for this data set, and thus fail to identify most of the reports that contain an abnormality. Two straightforward methods to be considered are keyword searches and International Classification of Diseases (ICD9) based searches. Because the reports are unlabeled, it is difficult for researchers to select reports that contain abnormalities in a specific region, e.g. The Audiological and Genetic Database (AudGenDB), a public, de-identified observational research database derived from EHR data sources, contains over 16,000 de-identified, unlabeled radiologist reports.

In audiologic and otologic research, the ability to use anatomic information described in radiology is essential to understand the causes of hearing loss for research subjects and to develop new treatment modalities. These methods have been applied to automate EHR text analysis in a variety of studies including phenotype extraction, adverse drug-event identification, and domain-specific radiology report classification.
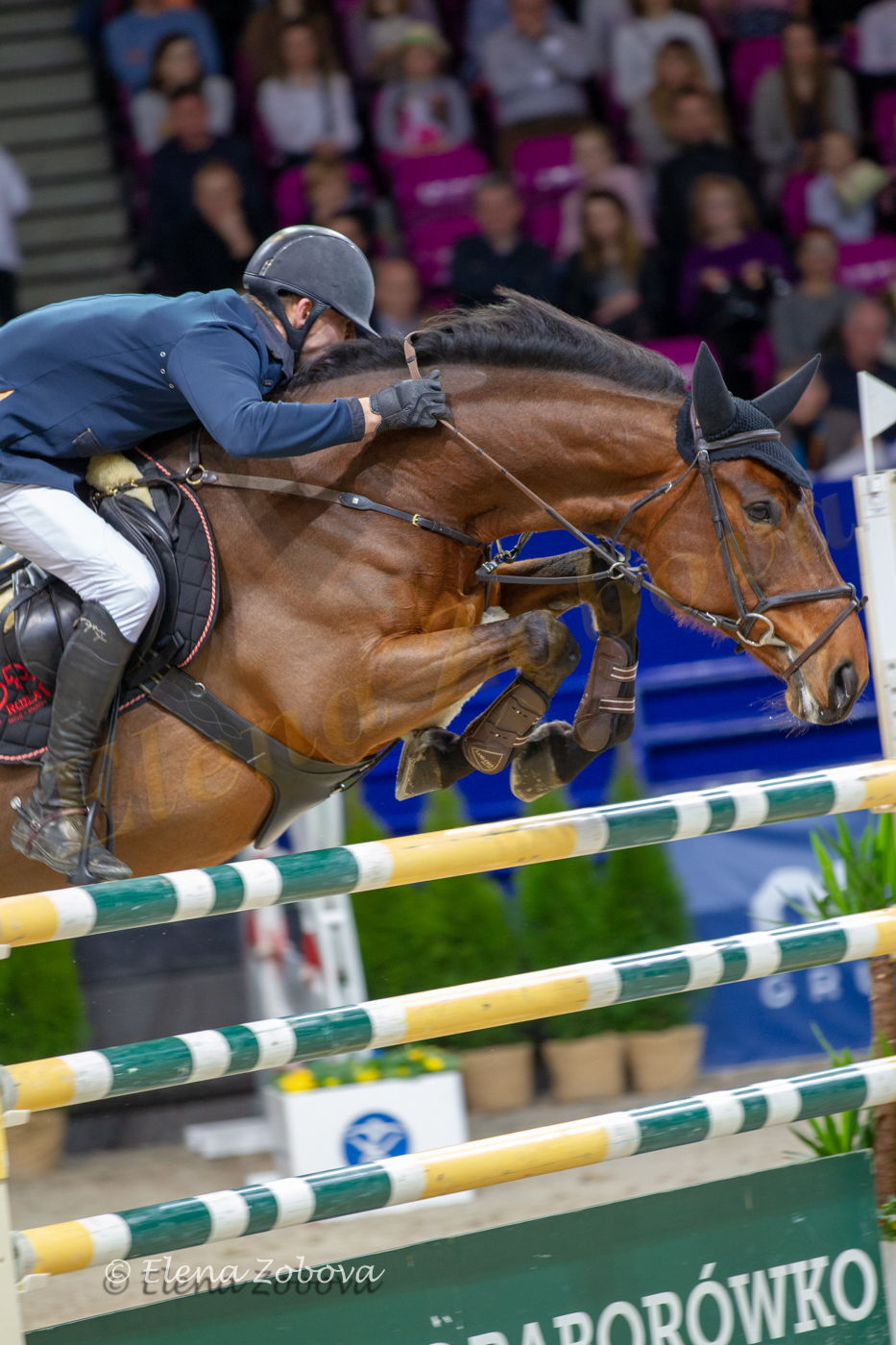
#Csillag kullo manual#
Natural language processing (NLP) and machine learning (ML) methods present an alternative to manual text review. Such manual review may be time consuming and expensive, particularly for large data sets. In the absence of automated processing, this requires trained data abstractors to manually review the text sources and identify discrete values of interest. Prior to research utilization, EHR text data, such as physician notes and radiology reports typically must be converted to discrete values, e.g. Electronic health records (EHRs) contain significant amounts of unstructured text that pose a challenge to their secondary use as a research data source.
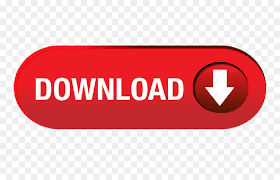